Yoshihiro Sato
Junior Associate Professor, Ph.D. in Engineering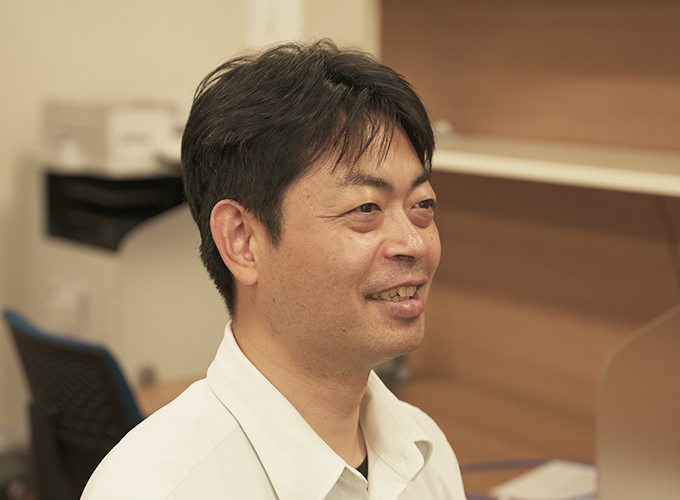
- sato.yoshihiro
- Areas of Research
- Robotics (Agricultural Robotics, Brain Computer Interface, Human-Robot Communication, etc.), Computer Vision (3D Digital Archiving), VR/MR(Realistic Tele-Communication, 3D HiFi Audio)
- Profile
- Research
-
Dr. Yoshihiro Sato obtained his Ph.D. in Engineering from the University of Electro-Communications in 2016, the same institution where he obtained his M.Sc. in 2001. In the time gap between his M.Sc. and Ph.D., he enrolled once for a doctoral program but retired. His unique career also includes working at an audio equipment company for three years, as well as conducting research at the Institute of Industrial Science at the University of Tokyo for 10 years before completing his Ph.D. on his second challenge.
Dr. Sato is currently engaged in the research of robotics and computer vision. At KUAS, he commenced researching a control system for a robot that aims to simplify operation by using computer vision and virtual relation. His research interests also include robotics with clear applications, such as in agriculture.
A chef at heart, Dr. Sato enjoys finding the next hidden gem in the restaurant scene. He also spends his spare time hiking to burn off those calories or taking in a live show.
-
Imitation Learning
The ability to learn from watching another person perform a task was a key development in human evolution. Today, this ability to learn from observation is recognized as a crucial evolutionary step in robotics programming. While attempts to train robots to learn from observation have come a long way since early efforts in the 1980s, recent developments in computers and optical devices are allowing for more practical applications.
The framework of “Learning From Observation” has three important components. The first is the observation of human or object behaviors in a task domain which we want to teach using a variety of sensors, especially optical devices, and the recording of that observation as data sequences. The next step is analysis. Robots, like humans, must be able to analyze data sequences and choose only the important pieces of information needed to describe the actions needed to perform the task. The final component is mimicry, where the robot must function to perform the task in a given environment or situation. Usually, the given environment is different from that where the behavior was observed, and the robot must account for this using the data it has gathered and analyzed. This is an especially challenging and interesting, aspect of teaching a robot to learn from observation.
On the other hand, in the case of animals and human beings, observing and mimicking is a very fundamental learning mechanism. As mentioned in the previous section, both humans and robots need to analyze data sequences, but in the case of humans and other animals, the analysis is unconscious. While in the case of robots, some kind of mathematical task model designed by humans has traditionally been required to correctly analyze the data and derive appropriate solutions. This lack of intuitive observation and learning ability is still a focus of robotics research today. However, robotics is now undergoing a drastic transition that may be just as important as the evolutionary step that humanity took when it began to learn from observation.
To give robots the ability to innately observe and learn, it is first important to observe the action of observing. When human beings are awake, we are always watching something consciously or unconsciously. How do we decide what to watch or ignore? Perhaps this is decided from a huge database of knowledge that we accumulate from the time we are born, or perhaps we are using a lot more than just our eyes to observe, such as our sense of hearing and touch. This is the topic that Dr. Sato is working on in his own research. In human language, we have many terms related to observation. For example, in English, we watch, see, look, and glance at things. In Japanese, there are also many expressions for observation: “見る”, “視る”, “観る”, “看る”, and so on. By creating a system that classifies the type of observation taking place, Dr. Sato hopes to create robots that engage in similarly innate observation, thereby bringing us one step closer to robots that can learn like us.